The misleading certainty of the coronavirus graphic
No COVID-19 map or graphic is as true as you want it to be
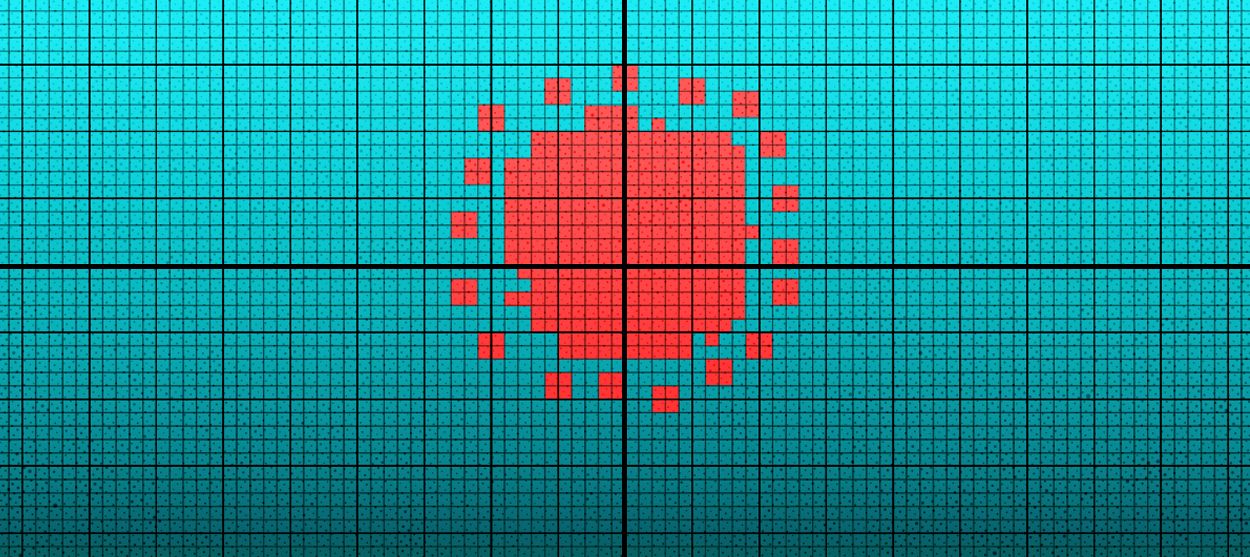
On Thursday morning, The New York Times published a map. Using anonymous cellphone data from millions of Americans, the map showed a county-by-county breakdown of "when average distance traveled first fell below two miles" since social distancing was advised to curb the spread of the novel coronavirus. The conclusion felt obvious: As one Times reporter summarized in a tweet he later deleted, "The South."
This map seemed to tell a simple, intelligible story, and that's precisely what we want from our pandemic data. We want — I know I want — to be able to say, "Here are the numbers of this pandemic. Here's what they'll do next. Here's what we can anticipate. Here's when this will end. Here is what is true."
But we can't do that. The data we have is conflicting, incomplete, and often misleading. Sometimes it measures the wrong thing. Sometimes the right thing is impossible to measure. Sometimes, even when the data is good, we aren't sure we can trust it — after all, we come into this pandemic less than four years after all our best data said Hillary Clinton would win the White House by a landslide. We come into it in the age of fake news, where even the meaning of the phrase "fake news" is disputed.
Subscribe to The Week
Escape your echo chamber. Get the facts behind the news, plus analysis from multiple perspectives.
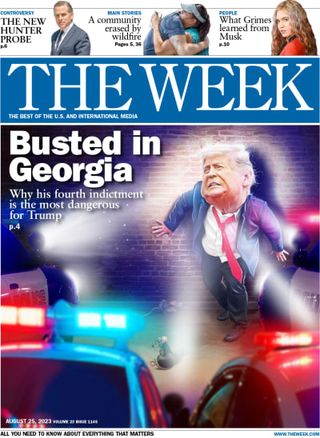
Sign up for The Week's Free Newsletters
From our morning news briefing to a weekly Good News Newsletter, get the best of The Week delivered directly to your inbox.
From our morning news briefing to a weekly Good News Newsletter, get the best of The Week delivered directly to your inbox.
We come into it in a state of enormous epistemological uncertainty while craving precisely the opposite. No coronavirus graphic is as true as we want it to be, but that want is so eager and the allure of cogent data visualization so strong that we can be all too willing to forget flimsiness of so much of the "truth" on offer.
The New York Times map is a good case study. The map, as widely shared, was not true. I'm not suggesting the Times presented any false information or willfully misled its readers. Rather, it was not true because — especially excerpted from its article for quick Twitter views — the map was so lacking in context it could not communicate anything like a complete truth. Compare, for example, that map to another one from the very same Times report:
Unlike the green and red map, which measures travel of a single, fixed distance of two miles, the grey and red map examines proportional change from normal travel. The South is still closer to normal than the Northeast, but the difference is significantly less. The first map's stark contrast is as much about differences of density — you typically have to go much farther to get groceries in rural Georgia than in Manhattan — as it is about differences of pandemic response. Thus, when we compare the green and red map to this CDC map marking places where more than 10 percent of the population lives at least a mile from the nearest grocery store and doesn't have a car, we find they have much in common:
The narrative the green and red map generated in isolation was deceptively straightforward and built on assumptions about the politics and culture of the South. A common suggestion I saw on Twitter — indeed one I made myself when I emailed the Times report to my husband Thursday morning — was that the red areas would correspond neatly to the red regions of a 2016 presidential election map. In fact, they don't.
Look for yourself: The Mountain West and the Rust Belt were Trump country in 2016, and they have exemplary performance in social distancing by the green and red map's measure (especially when you consider the extreme low density in great swathes of the West). And the rural South voted Trump in 2016, yes, but not as consistently as the western edges of Nebraska, Kansas, and Oklahoma, where residents stopped traveling distances of more than two miles quite early.
The narrative was wrong. Though it never lied, the green and red map didn't tell the truth — at least, not the easy, reliable truth we're seeking.
And the really unsettling thing is this map isn't an outlier. Lots of the data we're trading around — posting here and there in hope and dismay and glassy-eyed resignation — is similarly suspect, not because someone has been irresponsible or deceptive but because we just can't do better than that right now.
Every day, multiple times a day, I open my phone browser and type "co" in the URL field. I only have to type "co" to get the result I want because I have done this so often. My phone knows what I want when I type "co," which is this website running a daily, per-country tally of reported cases, deaths, and recoveries from the novel coronavirus. As of this writing, the United States has 228,727 total cases (of which 26,473 were diagnosed yesterday alone), 5,102 deaths, and 8,878 recoveries.
Or, at least, that's what this one website says. Johns Hopkins' ticker has a slightly lower total case count and a slightly higher death count, as does CNN's accounting, albeit with different figures. The CDC's case and death totals are both a bit lower than the site I check. The New York Times has different numbers still. I'll never know which is most correct.
And all five sites' figures are incomplete — dramatically so. The cases they report are only those with confirmed positive tests, and though it has accelerated, testing here in the U.S. remains insufficient and is rationed via guidelines that undoubtedly produce an undercount. Likewise, these death tolls are test-dependent, but studies of excess mortality (the number of deaths beyond a region's usual death rate) coming out of Europe suggest unidentified COVID-19 deaths may equal or exceed identified ones. And what about mild cases and asymptomatic carriers who never request a test at all? Asymptomatic carriers alone may represent as many as 50 percent of those who contract COVID-19 — but the rate of asymptomatic infection is another thing we don't know.
Death forecasts for the pandemic in its entirety have an even wider spread. Some suggest 100,000 to 200,000 Americans could die of COVID-19. Others say up to 2.2 million! As Maggie Koerth, Laura Bronner, and Jasmine Mithani write at FiveThirtyEight, that's "the difference between a death toll on par with the number of people who die from injury and violence annually in the U.S. and one that's closer to the number of people murdered when the Chinese communists moved to suppress counterrevolutionaries between 1950 and 1953. It is, in other words, the difference between a number we routinely live with, and one that changes a country forever."
There is no way to say with certainty which projection is correct, because each depends on an equation in which every component is itself in question: the rate at which the infection spreads, the accuracy of testing, the effectiveness of shelter-in-place orders and other mitigation efforts, the public's rate of compliance with those efforts, the availability and distribution of medical resources, the discovery of new treatment options, the development of a vaccine, the symptomaticity ratio, the effect of warm and humid weather — all of this and more is unknown or in flux or as-yet unmeasurable.
"All models are wrong," Mark Weir of Ohio State's ecology, epidemiology, and population health program told the FiveThirtyEight reporters. But, he added, the task for statisticians and scientists is "striving to make them less wrong and [more] useful in the moment."
We in the general public have a task too, which is to forge a path between suspicion and certainty, obsession and apathy, neither denying the reality that something very serious is happening nor hysterically seizing on all the worst predictions as proof of apocalypse.
It may be helpful to think of the data we have as the distorted little sketch you make as the timer relentlessly ticks in a game of Pictionary. It does not have the accuracy of a photograph. It might be so bad you have to scribble it out and start over. But it does have a basis in fact, in the prompt on your card, however poorly that prompt is reflected. The pandemic data we're getting can likewise give us a fluid, oft-revised idea of the truth, more impression than measurement, but hopefully enough to inform reasonable decisions and help us err on the side of prudence and care.
Sign up for Today's Best Articles in your inbox
A free daily email with the biggest news stories of the day – and the best features from TheWeek.com
Bonnie Kristian was a deputy editor and acting editor-in-chief of TheWeek.com. She is a columnist at Christianity Today and author of Untrustworthy: The Knowledge Crisis Breaking Our Brains, Polluting Our Politics, and Corrupting Christian Community (forthcoming 2022) and A Flexible Faith: Rethinking What It Means to Follow Jesus Today (2018). Her writing has also appeared at Time Magazine, CNN, USA Today, Newsweek, the Los Angeles Times, and The American Conservative, among other outlets.
-
Store closings could accelerate throughout 2025
Under the Radar Major brands like Macy's and Walgreens are continuing to shutter stores
By Justin Klawans, The Week US Published
-
Crossword: February 20, 2025
The Week's daily crossword
By The Week Staff Published
-
Sudoku hard: February 20, 2025
The Week's daily hard sudoku puzzle
By The Week Staff Published