How big data boosts discrimination
Algorithms can be prejudiced, too
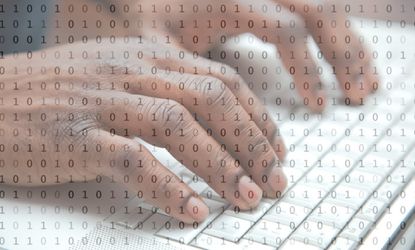
In 1977, the U.S. agency of Housing and Urban Development audited the real estate industry and discovered that African Americans were shown fewer properties (or told they were unavailable) and treated less courteously than white counterparts. Today, the Information Age has introduced modern discrimination problems that can be harder to trace: From search engines to recommendation platforms, systems that rely on big data could be unlocking new powers of prejudice. But how do we figure out which systems are disadvantaging vulnerable populations — and stop them?
Here's where it gets tricky: Unlike the mustache-twiddling racists of yore, conspiring to segregate and exploit particular groups, redlining in the Information Age can happen at the hand of well-meaning coders crafting exceedingly complex algorithms. One reason is because algorithms learn from one another and iterate into new forms, making them inscrutable to even the coders responsible for creating them, it's harder for concerned parties to find the smoking gun of wrongdoing. (Of course, sometimes coders or overseeing institutions are less well-meaning than others — see the examples to come).
So, how do we even begin to unravel the puzzle of data-driven discrimination? By first examining some of its historical roots. A recent Open Technology Institute conference suggested that high-tech, data-driven systems reflect specific, historical beliefs about inequality and how to deal with it. Take welfare in the United States. In the '70s, policymakers began floating the idea that they could slash poverty levels by getting individuals off welfare rolls. As part of that process, government computerized welfare case management systems — which would make it easier to track who was eligible to receive benefits and who should be kicked off. Today, these case management systems are even more efficient at determining program eligibility. The upshot? Computerized systems reduce caseloads in an increasingly black box manner. The downside: They do so blindly — kicking out recipients whether or not they're able to get back on their feet. That's contributing to greater inequity, not less.
Subscribe to The Week
Escape your echo chamber. Get the facts behind the news, plus analysis from multiple perspectives.
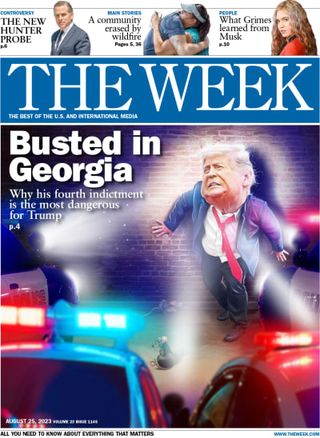
Sign up for The Week's Free Newsletters
From our morning news briefing to a weekly Good News Newsletter, get the best of The Week delivered directly to your inbox.
From our morning news briefing to a weekly Good News Newsletter, get the best of The Week delivered directly to your inbox.
That's not all, though. Even when systems are well-designed, it can be "garbage (data) in, discrimination out." A transportation agency may pledge to open public transit data to inspire the creation of applications like "Next Bus," which simplify how we plan trips and save time. But poorer localities often lack the resources to produce or share transit data, meaning some neighborhoods become dead zones — places your smart phone won't tell you to travel to or through, isolating these areas into islands of poverty.
Unfortunately, the implications of flawed data collection may not become apparent for years — after we have made policy decisions about our transit system, for example. Researchers refer to this issue of time as a sort of conditioning problem that arises from several different sources. In one case discussed, discriminatory conditioning happens because of the information itself. Take, for example, genetic information. In the U.S., police can collect DNA from individuals at point of arrest. This information identifies you much in the same way a fingerprint does. But your DNA also links you with others — your family members from generations before, relatives living today, and future generations. While it's hard to predict how law enforcement or others might use this information in the future, the networked nature of DNA makes it a high-risk candidate for implicating an entire group, and not just an individual.
In other cases, discriminatory conditioning happens because of the pervasiveness of collecting and sharing information, making it hard to control who knows what about you. Most Web pages regularly embed code that communicates to third parties to load an icon, cookie, or advertisement. Try searching for a disease — say AIDS — and click on a top result. Chances are the page will include icons for other applications not connected to the health site. The resulting effect — data leakage — is difficult to avoid: A Web page must communicate information about itself (e.g., "http://www…com/HIV") to icons so that the site loads correctly. That could be devastating for those who wish to conceal health conditions from data brokers or other third parties that might access and act upon your data profile.
Or consider the case of highly networked environments, where information about what you're doing in a particular space data gets sucked up, matched and integrated with existing profiles, and analyzed in order to spit back recommendations to you. Whether at home, out shopping, or in public, few people can be invisible. Homes come outfitted with appliances that sense our everyday activities, "speak" to other appliances, and report information to a provider, like an electric utility company. While it's presumptuous to say that retailers or utility companies are destined to abuse data, there's a chance that information could be sold down the data supply chain to third parties with grand plans to market predatory products to low-income populations or, worse yet, use data to shape rental terms or housing opportunities. What it boils down to is a lack of meaningful control over where information travels, which makes it more troublesome to intervene if and when a problem arises in the future.
Sign up for Today's Best Articles in your inbox
A free daily email with the biggest news stories of the day – and the best features from TheWeek.com
So what's possible moving forward? Waiting is definitely not the answer. With collective and personal control, autonomy, and dignity at stake, it would be wrong to leave governments or industry to respond to problems without independent research input. A relatively simple strategy would be to ensure collaboration and coordination between social and computational research. There's also much to be done in terms of gaining greater access to datasets which various laws otherwise impede (e.g., computer fraud and abuse, intellectual property or trade secrets). Crowdsourcing the discovery of data-driven discrimination is another possibility, where like the HUD audits, users that are similar on all but one trait monitor and report experiences with a variety of automatable systems.
Trying many approaches, and testing them out now may seem like an ambitious agenda, and it is. But in a period of such uncertainty — about how laws, market practices, social norms and practices, or code can safeguard collective and personal dignity, autonomy, and rights — experimentation and iteration is critical to exposing harm or benefit. Only then will we generate stories and evidence rigorous enough to reveal discrimination when it happens.
But for now, that uncertainty can't get resolved quickly enough as we head into an era of more and more data collection, analysis, and use. There's a real threat that things are going to go badly, and disproportionately burden the poorest and most marginalized among us. The twin dynamics will only accelerate the divide. Despite the complexity of this task, the time to confront data-driven discrimination is now.
Sign up to get The Weekly Wonk, New America's digital magazine, delivered to your inbox each Thursday here.
More from The Weekly Wonk...
Create an account with the same email registered to your subscription to unlock access.
-
In what countries is assisted dying legal or in consideration for being made legal?
In the spotlight More countries are granting more people the right to die
By Devika Rao, The Week US Published
-
5 captivating books to read in May
the week recommends Brittney Griner tells her own story, a coming-of-middle-age novel and more
By Theara Coleman, The Week US Published
-
The secretive practice of 'catch-and-kill' tabloid journalism
The Explainer Outlets such as the National Enquirer have become infamous for using the practice
By Justin Klawans, The Week US Published